Unlocking Business Potential with Image Data Annotation
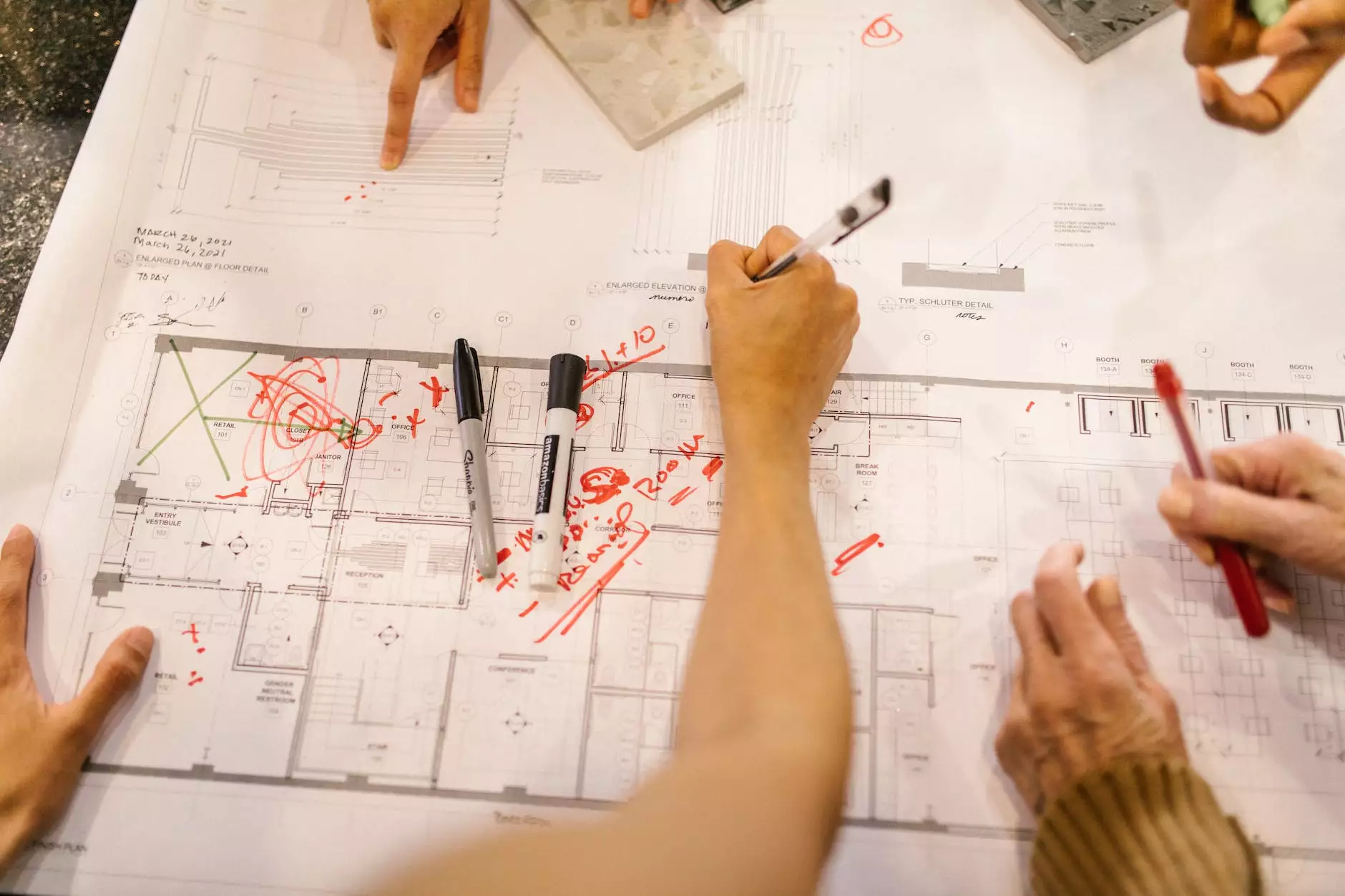
Image data annotation is revolutionizing modern business practices by providing the essential tools to leverage the immense potential of artificial intelligence (AI) and machine learning (ML). In today's competitive landscape, organizations aim to use data-driven insights to enhance decision-making, drive growth, and improve customer satisfaction. This article delves into the intricacies of image data annotation, its critical role in various industries, and how businesses can benefit significantly from implementing robust data annotation solutions such as those offered by keylabs.ai.
Understanding Image Data Annotation
Image data annotation refers to the process of identifying and labeling images to provide context and meaning to the data. This process is fundamental for training AI models, particularly in the fields of computer vision and natural language processing. By annotating images, businesses can teach AI systems to recognize patterns, objects, and anomalies, enabling applications in various sectors including healthcare, automotive, retail, and more.
The Importance of Image Data Annotation
In a world dominated by visual content, the significance of image data annotation cannot be overstated. Here are some key reasons why:
- Enhancing AI Performance: Accurate image annotations are crucial for training high-performing AI models. The more precise the annotations, the better the model's predictions will be.
- Improving Automation: Automated processes driven by AI can significantly improve operational efficiency, reducing human error and cutting labor costs.
- Driving Innovation: Data analytics supported by image annotations can lead to innovative solutions in product development and service delivery.
- Boosting Customer Experience: In e-commerce, for example, well-annotated images can enhance search functionalities, helping customers find exactly what they're looking for.
Applications of Image Data Annotation in Various Industries
The applications of image data annotation are vast and varied, touching virtually every industry. Below are some notable examples:
1. Healthcare
In healthcare, image data annotation plays a pivotal role in diagnostics and treatment planning. AI algorithms trained on annotated medical images, such as X-rays, MRIs, and CT scans, can assist in detecting diseases more accurately and quickly.
Examples:
- Radiology: Annotated medical images help radiologists identify tumors and other abnormalities.
- Pathology: AI models can analyze annotated slides to assist pathologists in diagnosing conditions like cancer.
2. Automotive
The automotive industry is rapidly adopting image data annotation in the development of autonomous vehicles. Datasets that include annotated images enable AI systems to recognize road signs, pedestrians, and obstacles, enhancing the safety and reliability of self-driving technology.
Examples:
- Object Detection: Cars must be trained using annotated images to consistently identify and react to other vehicles, cyclists, and pedestrians.
- Road Condition Assessment: Data annotation assists AI in interpreting road conditions and adjusting driving behavior accordingly.
3. Retail
In retail, image data annotation helps businesses refine their marketing strategies and improve customer experiences. Annotated images enhance product visibility and aid in inventory management.
Examples:
- Visual Search: Retailers can implement visual search functionalities powered by AI that utilize annotated product images to enhance search results.
- Inventory Automation: Annotated images facilitate automated inventory checks, helping retailers manage stock more efficiently.
Choosing the Right Data Annotation Platform
To successfully harness the benefits of image data annotation, businesses must select the appropriate data annotation platform. Here are key considerations to keep in mind:
1. Scalability
Choose a platform that can easily scale according to your data needs. As your business grows, your data volume will likely increase, and your annotation tools must keep pace without compromising quality.
2. Accuracy and Quality Control
The accuracy of annotations directly impacts the performance of AI models. Hence, it's vital to choose a platform that employs rigorous quality control measures, including machine learning-assisted reviews and human validation.
3. Ease of Integration
Look for solutions that seamlessly integrate with existing technologies and workflows. A smooth integration ensures that your team can adopt the new platform without disruption.
4. Cost-Effectiveness
Analyze the pricing structure of the data annotation platform. It's essential to balance cost with quality and service before making a commitment.
Best Practices for Effective Image Data Annotation
Implementing best practices in image data annotation can significantly enhance the quality and effectiveness of your data sets. Consider the following:
1. Define Clear Objectives
Before beginning your annotation project, clearly define your objectives. Specify what you hope to achieve, whether it’s improving model accuracy or enhancing customer insights.
2. Standardize Annotation Guidelines
Creating standardized guidelines for annotators ensures consistency across the dataset. Clear definitions of labels and instructions prevent ambiguity and confusion.
3. Utilize Both Manual and Automated Annotations
Combining manual and automated annotation can enhance efficiency. Use automated tools for preliminary annotations and allow human annotators to refine results for accuracy.
4. Regularly Review Quality
Continuous monitoring and reviewing of the annotation quality can help identify and rectify issues early. Implement a feedback loop where annotators can learn from mistakes.
Case Studies of Successful Image Data Annotation
Case studies provide concrete examples of how image data annotation drives substantial business benefits. Here are a couple of noteworthy instances:
1. Healthcare AI Innovations
A major healthcare provider partnered with a data annotation platform to annotate thousands of medical images for training their diagnostic AI models. As a result, they saw a 25% increase in diagnostic accuracy, allowing them to expedite treatment plans and improve patient outcomes.
2. Autonomous Driving Development
An automotive company focused on developing self-driving technology utilized a comprehensive data annotation service to label millions of road images. This investment enabled their vehicles to achieve industry-leading object detection capabilities, enhancing safety and instilling confidence in their self-driving features.
Conclusion: The Future of Business with Image Data Annotation
As technology evolves, the role of image data annotation will only become more integral in shaping the future of business across various sectors. Embracing these techniques not only empowers AI and automation but also drives enhanced customer experiences and operational efficiencies. Companies that recognize and leverage the power of image data annotation today will undoubtedly maintain a competitive advantage in the rapidly developing digital landscape.
To explore innovative data annotation tools and platforms that can take your business to the next level, visit keylabs.ai and discover the capabilities that await!